In the rapidly evolving landscape of technology, Vision AI stands out as a transformative force, particularly in the realm of enterprise operations. Vision AI, or visual artificial intelligence, leverages advanced machine learning and computer vision techniques to analyze and interpret visual data. This powerful technology is increasingly being integrated into enterprise systems, driving significant value across various dimensions. Vision AI can optimize processes, enhance quality assurance, improve product quality, streamline maintenance, and enable more effective decision-making. Here’s a detailed look at how Vision AI can create substantial business value at the enterprise level.
The integration of Vision AI into enterprise systems brings several compelling benefits:
- Enhance Human Capability: Vision AI augments human capabilities by automating routine visual tasks, allowing employees to focus on more complex and value-added activities.
- Higher Quality: Automated visual inspections and analyses conducted by Vision AI systems are often more consistent and accurate than manual processes, leading to improved product and service quality.
- Faster Decision Making: Vision AI enables real-time data analysis and insights, significantly speeding up the decision-making process and enhancing operational efficiency.
- Process Optimization: By providing detailed insights into operations, Vision AI can identify bottlenecks and inefficiencies, allowing enterprises to optimize their processes and improve overall productivity.
- Maintenance: Vision AI can predict equipment failures and maintenance needs by analyzing visual data, reducing downtime and extending the lifespan of machinery.
- Standardization of operations, by implementing technologies that can make decisions (instead of humans)
- Addresses the shortage of skilled employees
Join our upcoming event about the value of Vision AI at the enterprise here
For Vision AI to deliver real business value, it must be integrated seamlessly into the broader enterprise system. Integration is key to ensuring that the insights derived from visual data are utilized effectively across various business functions. This comprehensive integration process involves aligning Vision AI with existing workflows, systems, and business strategies to maximize its impact.
When Vision AI is fully integrated, it becomes a core component of the enterprise system, driving substantial business value. This integration ensures that visual data insights are effectively utilized across various business functions, such as quality control, supply chain management, and customer service. For example, a manufacturing enterprise might integrate Vision AI with its SAP enterprise resource planning (ERP) system to monitor production quality in real-time, instantly alerting managers to any defects or deviations from standards. This not only enhances product quality but also reduces waste and optimizes resource use.
On the other hand, a standalone Vision AI system, without proper integration, may fail to deliver significant value. If Vision AI operates in isolation, its insights might not be fully leveraged, leading to missed opportunities for optimization and improvement. Therefore, it’s essential that Vision AI is not isolated but part of a comprehensive enterprise strategy. This involves working closely with IT departments and solution providers like SAP to ensure seamless integration and interoperability with other enterprise systems.
Moreover, solutions from companies like SAP can facilitate this integration, providing a comprehensive platform that supports Vision AI. SAP’s integrated solutions can help businesses unify their Vision AI initiatives with other enterprise functions, ensuring that visual insights contribute to broader business objectives.
Implementing Vision AI involves several key components:
Hardware: The physical enablers of Vision AI, such as cameras and servers from Lenovo, are critical for capturing and processing visual data.
Services: The development of specific features and functionality tailored to the enterprise’s needs is crucial for the success of Vision AI. This includes everything from initial setup to ongoing support and enhancements.
Vision AI Core Components: The trained AI models form the core of the system, constantly improving with use and becoming more accurate over time.
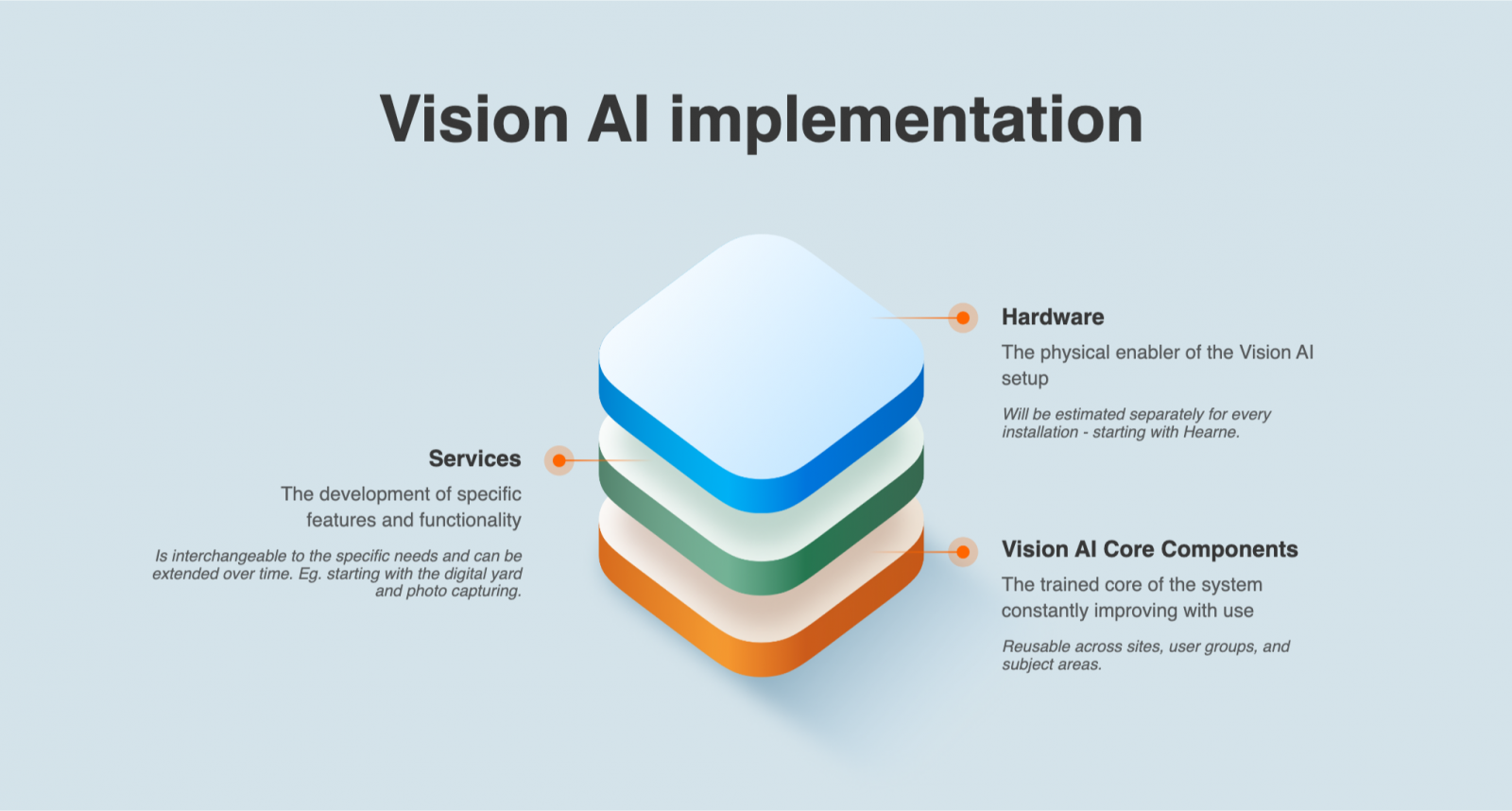
The journey of integrating Vision AI into an enterprise begins with a well-defined process that ensures the technology is both effective and aligned with business goals. This process typically involves several critical stages:
Acquire Data: The foundation of Vision AI is high-quality data. Enterprises collect data through cameras and synthetic data generation methods, ensuring a robust dataset for training AI models.
Annotate: The collected data is then meticulously annotated with accurate labels that reflect the business objectives. This step is crucial for training the AI model effectively.
Model Training: Leveraging server-grade GPUs from industry leaders like Nvidia, the annotated data is used to train the AI model. This step involves iterative testing and validation to achieve the desired accuracy and performance.
Deploy: Once trained, the model is deployed into the production environment through a DevOps pipeline. Enterprise solutions from companies like Lenovo provide the necessary infrastructure for smooth deployment and scalability.
Detection: The deployed model becomes live and begins running detection tasks, performing actions that drive business value.
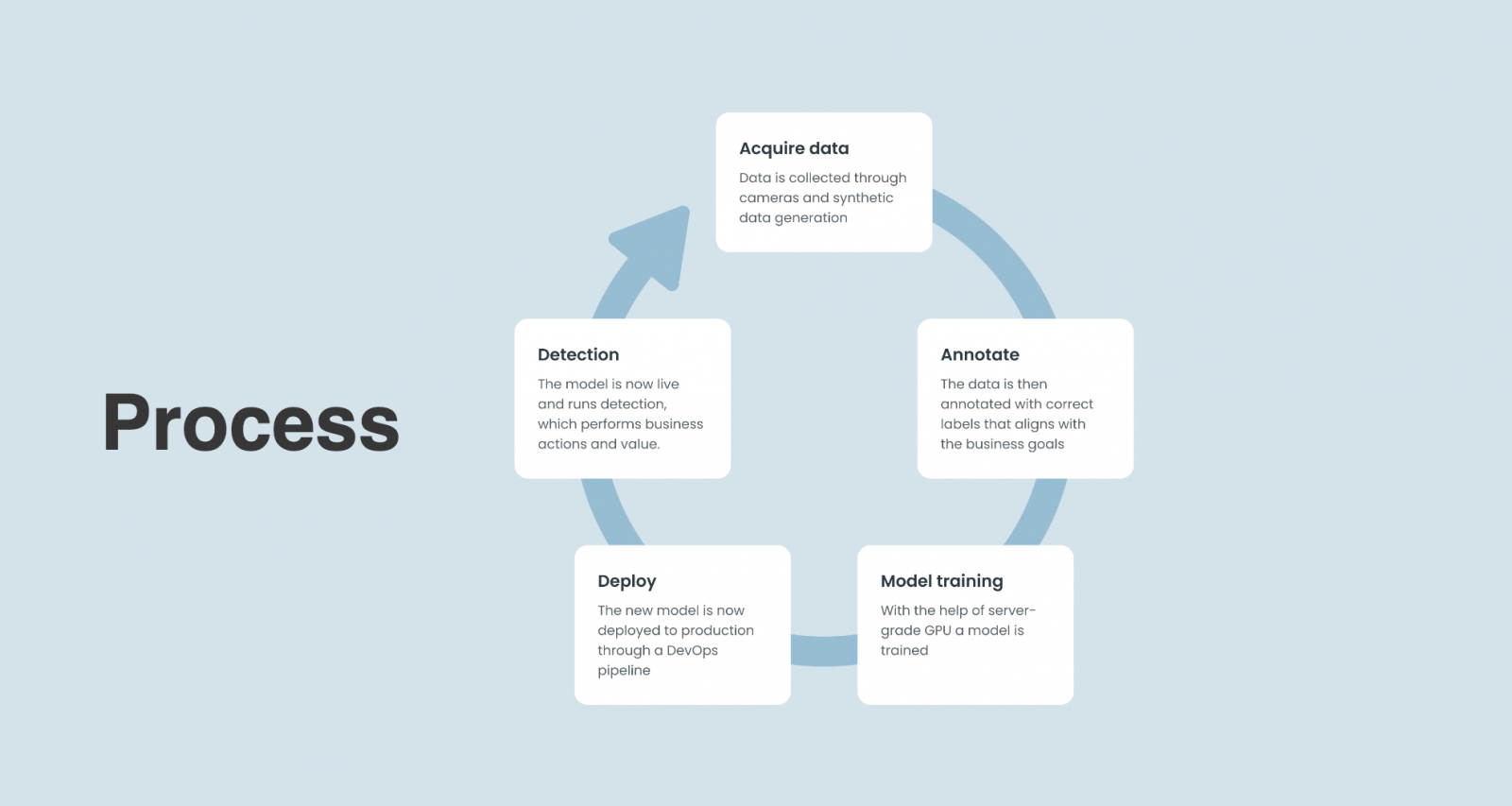
Implementing Vision AI in an enterprise follows a structured timeline, ensuring a seamless transition from concept to deployment:
Week 1: Workshop: The initial phase involves workshops to clearly define the objectives and scope of the Vision AI project.
Week 3: First Model Development: Early model developments are made collaboratively, involving quality assurance (QA) to ensure alignment with business quality standards.
Week 3-14: AI Development: This phase focuses on capturing high-quality training data, manual verification, and annotations, which are essential for developing a robust Vision AI model.
Week 14: AI Deployment: The deployment phase involves integrating the AI model into the production environment, ensuring it can process real data effectively.
Week 22+: Continuous Model Development: Post-deployment, the focus shifts to continuous model development, where new data is used to refine and enhance the model, ensuring it remains relevant and accurate.
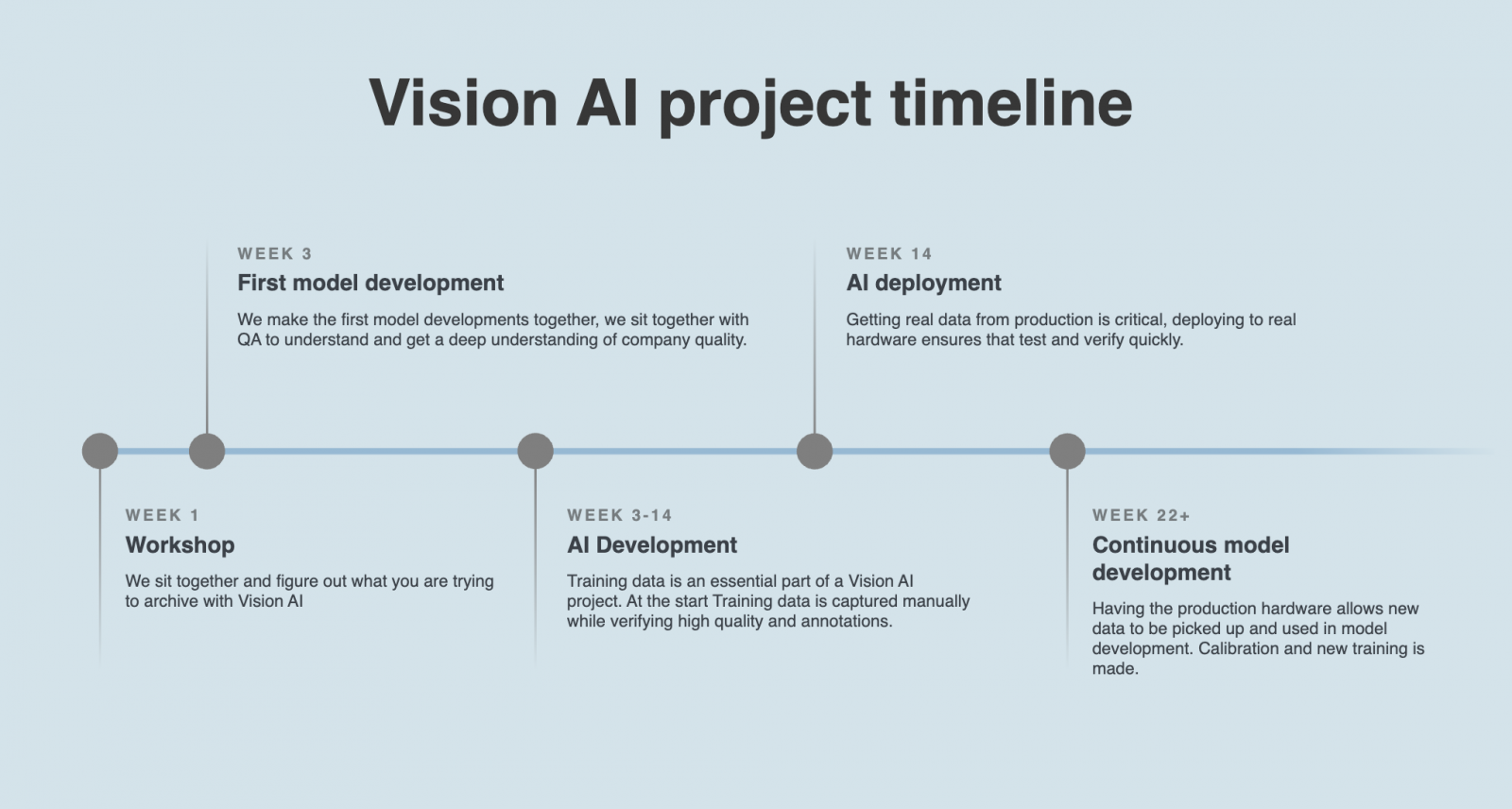
Vision AI represents a significant technological advancement with the potential to revolutionize enterprise operations. By following a structured implementation process, integrating Vision AI into broader enterprise systems, and focusing on continuous development and improvement, businesses can unlock substantial value. Enhanced human capabilities, higher quality outputs, faster decision-making processes, optimized operations, and predictive maintenance are just a few of the many benefits Vision AI brings to the enterprise, driving growth and competitive advantage in an increasingly data-driven world. Leveraging technologies from Nvidia, Lenovo, and SAP can further enhance the effectiveness and integration of Vision AI, ensuring it delivers maximum value to the enterprise.
Join our upcoming event about the value of Vision AI at the enterprise here